AI in Medicine: A Breakthrough or an Overhyped Experiment?
Reviewing the Superhuman Performance of a Large Language Model in Physician Reasoning
The intersection of artificial intelligence (AI) and medicine has long been a subject of fascination. Still, a recent study, Superhuman Performance of a Large Language Model on the Reasoning Tasks of a Physician by Brodeur et al. (2024), takes this discussion to a new level. Published in The Lancet, this study claims that OpenAI’s o1-preview large language model (LLM) surpasses human physicians in complex diagnostic reasoning tasks.
But is this truly a game-changer for healthcare? Or does it merely demonstrate AI’s ability to excel in structured test conditions while struggling with real-world complexity? This blog delves into this significant study’s findings, strengths, limitations, and future implications.
The Study at a Glance
Brodeur et al. (2024) evaluated o1-preview’s performance on five key clinical reasoning tasks:
The model’s outputs were compared against previous AI models (including GPT-4) and hundreds of real-life physicians, including medical students, residents, and attending doctors.
Key Findings
Key findings from the articles are:
The authors argue that o1-preview’s superior performance demonstrates AI’s potential to enhance clinical decision-making. However, they also caution against over-reliance on AI without real-world trials and regulatory oversight.
Visualizing AI’s Progress in Diagnostic Accuracy
The study by Brodeur et al. (2024) compares the o1-preview’s performance to other diagnostic tools and clinicians using NEJM Clinicopathologic Conferences (CPCs) as a benchmark. The figure below highlights the percentage of correct diagnoses included in the differential diagnosis for various tools and models tested between 2012 and 2024.
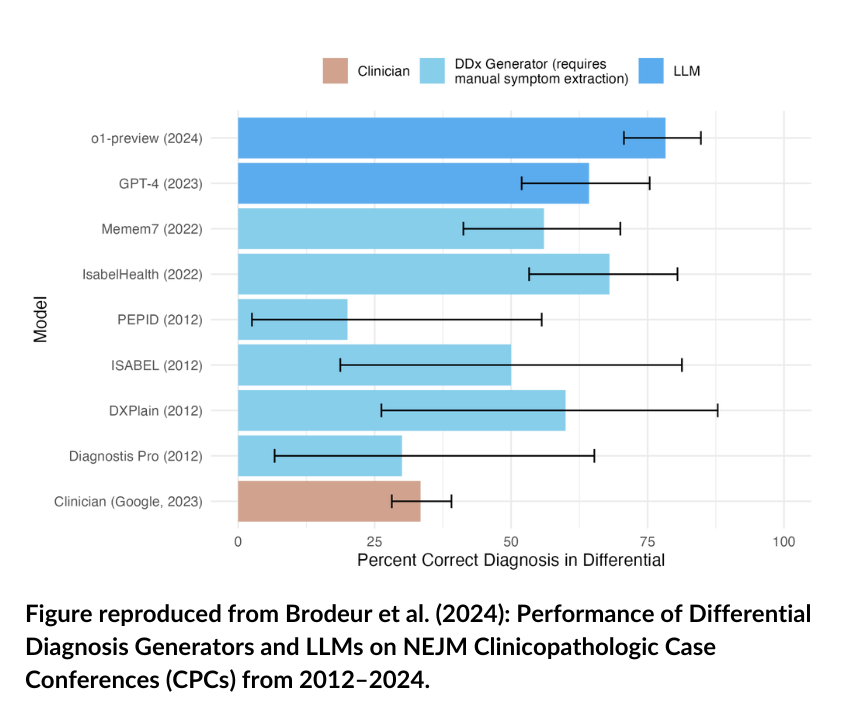
The above visual underscores three significant trends:
- Steady Improvement Over Time: Diagnostic tools, particularly LLMs, have consistently improved in accuracy since 2012.
- Superhuman Performance: o1-preview surpasses human clinicians (Google 2023) and earlier AI models like GPT-4 in identifying correct diagnoses.
- The Decline of Traditional Tools: Legacy diagnostic platforms (e.g., ISABEL and PEPID) lag far behind newer AI models, reinforcing the shift toward LLM-based solutions.
go to: | Start | Top of This Section |End
Comparing AI and Human Performance
The study also evaluated the performance of o1-preview in comparison to GPT-4 and physicians on two critical tasks:
- 1
Management Reasoning – Using Grey Matters Management Cases, o1-preview significantly outperformed GPT-4 and human physicians (with or without supplementary resources). The graph below shows its dominant scores across all metrics.
- 2
Diagnostic Reasoning – For Landmark Diagnostic Cases, o1-preview matched GPT-4 and physician performance, with no statistically significant differences observed. This suggests parity between current LLMs and trained professionals in structured diagnostic tasks.
A comparison of AI and Physician Performance is depicted in the plot below.
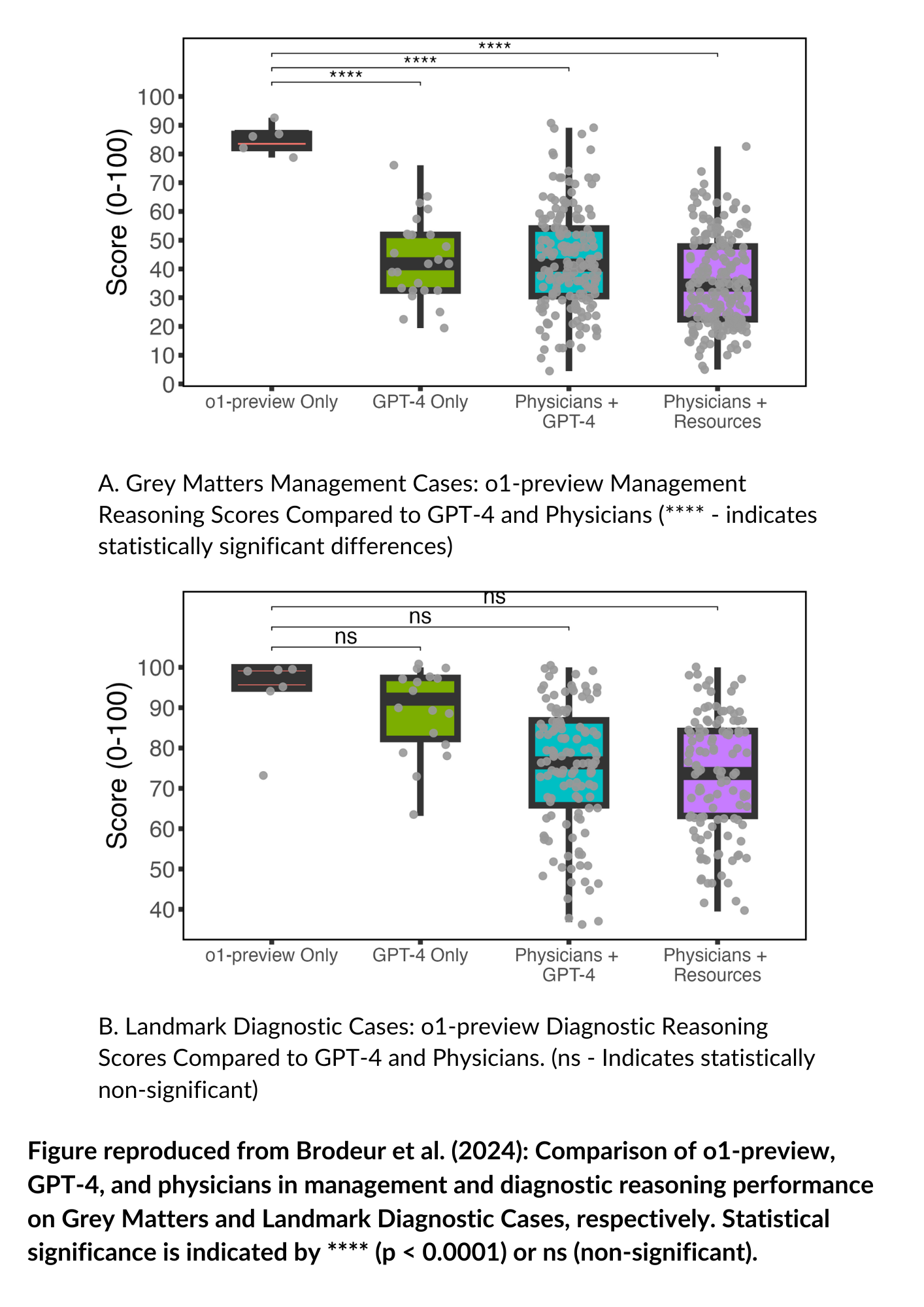
The above visual underscores:
- o1-preview has a clear advantage in management reasoning, likely due to its ability to synthesize guidelines and prioritize treatment decisions.
- The consistency across diagnostic reasoning tasks, indicating that AI and physicians may complement one another in such scenarios.
go to: | Start | Top of This Section | End
The Pros: Why This Study Matters
If integrated into electronic health record (EHR) systems, such AI models could help physicians focus on critical decision-making and patient interactions rather than administrative burdens.
go to: | Start | Top of This Section | End
The Cons: Where AI Still Falls Short
However, the question of how such a regulatory system would be monitored or enforced remains open, particularly as AI tools gain widespread, organic adoption among clinicians and the public without formal oversight. While Brodeur et al. (2024) focus on evaluating AI’s diagnostic reasoning capabilities, the study does not extend to discussions on the ethical or regulatory implications of AI-driven medical decision-making. As AI integration into healthcare progresses, questions around liability, fairness, and governance will need to be addressed by policymakers and regulatory bodies.
go to: | Start | Top of This Section | End
Future Implications: What’s Next for AI in Medicine?
go to: | Start | Top of This Section | End
Final Verdict: A Promising Step, But Not a Replacement for Doctors
The study by Brodeur et al. (2024) is a milestone in AI-driven medical reasoning, but it is not a definitive breakthrough for clinical practice—yet!
Bottom Line:
The shift toward AI-human collaboration in healthcare has already begun, but its evolutionary path and final destination remain uncertain. As AI models like o1-preview continue to improve, the question is no longer if AI will be integrated into medical practice—but how.
What Do You Think?
Please email your thoughts to [email protected] or scroll down and leave us a comment below.
go to: | Start | Top of This Section | End
References
Brodeur, P.G., Buckley, T.A., Kanjee, Z., Goh, E. and Rodman, A. (2024) ‘Superhuman performance of a large language model on the reasoning tasks of a physician’, The Lancet. Available at: https://doi.org/10.48550/arXiv.2412.10849 (Accessed:2-February-2025 ).
McDuff, D., Raghavan, P., Liang, P., Ong, E., D’Amour, A., Ramaswamy, S., Kelly, C.J. and Kornblith, S., 2023. Towards accurate differential diagnosis with large language models. arXiv preprint arXiv:2312.00164. Available at: https://arxiv.org/abs/2312.00164 (Accessed:3-February-2025).
go to: | Start | Top of This Section | End
Leave A Comment